What Are The Potential Challenges Of Implementing Artificial Intelligence In SEO?
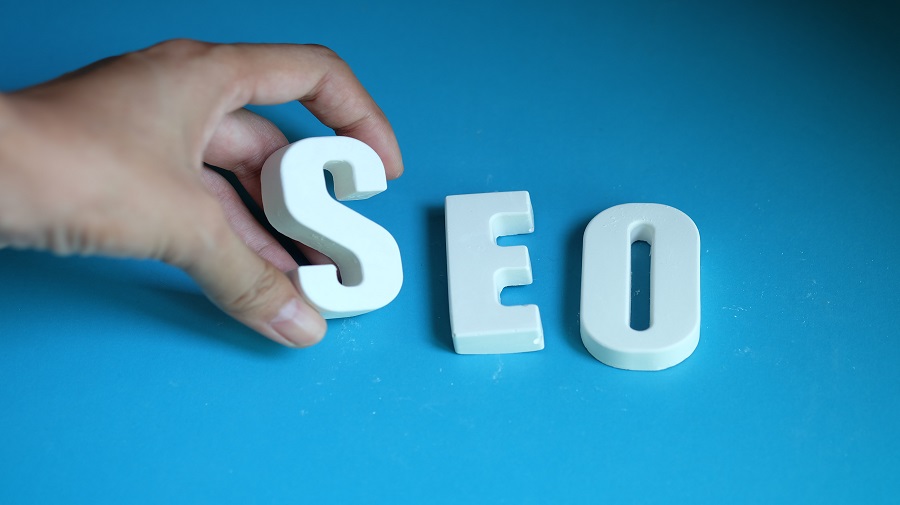
AI is getting into almost everything, it has lots of benefits, but eventually, AI has no brain, and it cannot offer what is not fed into it. Plus, there are a lot of loopholes in how AI systems work. From outdated algorithms to misinterpreting what humans really search for, AI still needs help getting it right. If we talk about SEO, AI is capable of automating many tasks and helping with efficiency. Still, there are some challenges that hinder its implementation. Let’s break down those AI weak spots and find better solutions.
What are some challenges associated with the Implementation of AI in SEO?
————————————
AI Can Provide Misleading Information
You might have used AI for different SEO tasks, including keyword research, content creation, technical SEO optimization, data analysis, and competitor analysis. Here’s how you likely employ it:
- Conduct extensive search data to identify relevant keywords, uncover emerging trends, and propose content ideas.
- Created headlines, product descriptions, meta tags, and even entire articles.
- Identify and address issues such as broken links, slow page load times, and crawl errors that could hinder search engine rankings. Conduct data analysis, providing valuable insights into website performance, user behavior patterns, traffic sources, and areas for improvement.
- Conduct competitor analysis by monitoring rivals’ strategies, pinpointing their strengths and weaknesses, and enabling businesses to refine and adapt their SEO approach accordingly.
However, were you confident in deploying AI without verifying it? Could you fully trust its output? If you did check the results thoroughly, you might have observed the mistakes mentioned below.
1. Irrelevant or Outdated Keywords
According to SEO Toronto Experts, relevance is subjective, varying among users. AI may struggle to capture these nuances accurately. Search engines personalize results based on factors like location and search history, which AI may not consider. AI may generate keywords from visual or auditory content, which may not align with the user’s query. Lastly, technical issues like broken links or indexing errors can affect keyword relevance, as can bugs or glitches in the AI system.
2. Factual Errors in Content
AI models are trained on limited or outdated code examples, it may fail to understand the modern syntax. Also, it can misinterpret the prompts sometimes and address the task incorrectly. In code-based inputs, AI may present you with code using outdated libraries.
3. Missed Technical SEO Issues
AI algorithms may not be designed to detect all potential technical issues, especially those requiring human interpretation or understanding of specific website contexts.
4. Misleading Data Interpretation
AI dashboards can present data in visually appealing ways, but without careful analysis, there’s the risk of drawing superficial conclusions or overlooking trends that a human expert would catch.
5. Inaccurate Competitor Assessments
AI might misinterpret competitor tactics, prioritize metrics that matter less, or fail to spot emerging strategies used by smaller, more agile rivals.
But, still, you can automate a lot of your tasks to save your time and energy. You just need to be careful.
Overcoming the Challenges in AI Development
————————————
A. Data Quality and Verification
In the area of artificial intelligence (AI), where the changing environment is dynamic, the basis of the success of model development comes from the quality and accuracy of the data used for training. Garbage in, garbage out – this saying reflects the real situation in the domain of AI since the productivity of models is directly proportional to the quality of data they are studied on.
Data quality is by no means a technical issue. It is a strategic issue. For the AI models to be reliable, there is a need to ensure a process of rigorous data verification is in place. It includes the use of data-validation tools of the latest sophistication with the introduction of human verification. Humans give their knowledge and operate in context, allowing them to spot those details that can be overlooked by automated systems.
Situations where errors have large impacts require tight integration of human oversight. In domains that account for health services, finance, or automotive technologies the substitution by a self-reliant verification procedure can open a path in the way of major risks. Hence, organizations have to tap the efficiency of AI systems while employing human judgment to check on the quality and reliability of the outputs.
B. Understanding Context and Nuance:
Although AI has won the battle in the majority and is becoming better, it still can’t deal with the complexities of understanding the human mind and behavior. Human behavior is complex, responding to a wide variety of stimuli comprising cultural influences, prior experiences, and emotional states.
It cannot be denied that AI still has limitations in grasping the subtleties of the situations. AI should be considered as a ‘helper’ rather than a ‘replacement’ by organizations. The union of AI insights and human abilities is going to release the predominant power. Human creativity, intuition, and the ability to get rapidly the figurative and contextual meanings are a notch above all and, thus, provide necessary refining for AI outputs.
Inter-disciplinary cooperation becomes more important, building a platform where data scientists, engineers, and domain experts collaborate. By collaborating the precision of AI algorithms with the intuition of human experts, organizations can construct more resilient models that are inclusive of the complexities of human behavior as well as the reasoning behind it.
C. Continuous Monitoring and Improvement:
AI development is not terminated with model deployment, but it is just the beginning of a continuous loop for model monitoring and improvement. The data’s dynamism and the changing user behavior terrain compel continuous alertness. Organizations need to provide a permanent monitoring mechanism of AI performance in order to maintain adequate goals and keep the models on track.
Continuous AI algorithm updates and robust training data are inevitable as new patterns emerge, bias is corrected, and overall performance is enhanced. This continual approach not only updates AI systems to stay relevant but also to meet the dynamic demands of users. A proactive approach to anticipating probable problems and reacting fast by adjusting models depending on real-world results is an important factor in ensuring the continuing effectiveness and stability of AI systems in the long run.
Ethical dimensions and bias in the Artificial Intelligence development process.
————————————
AI algorithms replicate biases present in the training data. This can lead to discriminatory outcomes in areas like:
Keyword suggestions: AI could suggest keywords that shall be built upon belittling/biased ideas or accommodate search queries that are borderline offensive.
Content generation: The unintentional reflection of the biases contained in the training data of AI-generated content may result in such use of questionable terms as unfair and offensive language.
Personalization: AI-based search findings have the potential of being biased by user demographics, past behavior, or other parameters, so it could be one of the reasons for the narrow scope of the search in terms of information diversity.
It’s crucial to address these ethical concerns by:
- Promoting data diversity and fairness: Make sure that data used for training is representative of different demographics and views.
- Implementing bias detection and mitigation techniques: How to validate AI models for potential biases and put some protections in place in order to avoid discriminatory outcomes?
- Maintaining human oversight and accountability: Human beings ought to be involved in the AI development, implementation, and monitoring processes to make sure that it is used ethically.
Sharing the Ethical Challenges and Bias of AI Technology Development
The best AI systems on the market are not safe from the biases embedded in the data they are trained on. This consideration brings ethical issues to the foreground as AI algorithms have the capacity to do nothing but replicate and worsen already existing biases, with discriminatory results in their practical implications.
One instance where bias can show up is in keyword recommendations. If the AI algorithm recommends keywords, which may be in an accident, it could spread harmful stereotypes and promote discriminatory search queries. The implications of these biased keyword recommendations are critical as they distort the information and can cause changes in users’ perceptions and maintain societal stereotypes.
To address these ethical concerns, it is imperative to implement proactive measures:
Promoting data diversity and fairness: Assuring that the training data, which AI models use, is comprehensive and inclusive is necessary. This involves taking into account a number of demographic criteria, perspectives, and cultural nuances in the data so as to eliminate the possibility of propagating biases.
Implementing bias detection and mitigation techniques: Frequently auditing AI models for potential biases and also appropriately putting in relevant safeguards is very vital. This entails regular monitoring and evaluation to uncover any disparities, hence fixing the problems in a timely manner.
Maintaining human oversight and accountability: It is humans who should play the central part of the whole process from the commencement of AI development. From inception to realization and feedback, human supervision safeguards ethical and responsible application. Humans can provide background information, use nuanced angle,s and interfere in the event of biased results.
Conclusion
Undoubtedly AI is a powerful task that can enhance the efficiency but human intervention is must to get the work done in right way.