What is Metadata Management? The best Metadata Management Tools
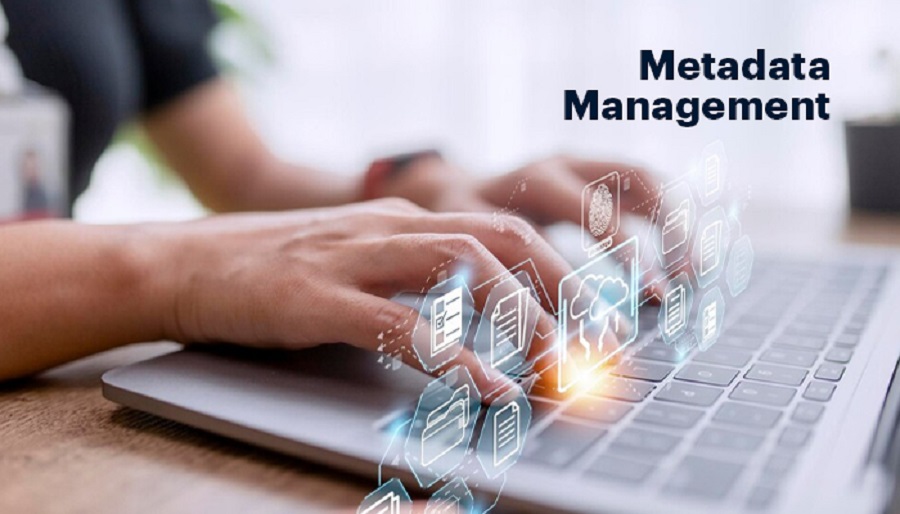
Metadata management is an agreement between organizations to define information assets to transform data into enterprise assets. As the amount and variety of data grows, metadata management becomes even more important to extract business value from vast amounts of data. Metadata management starts with properly defining metadata. Some people call metadata “data about data”, but that’s only part of the picture.
Metadata is actually more than just a description of the data. Metadata assumes new roles every day as data complexity increases. In some cases, the metadata may be relevant to the business perspective of quarterly sales figures. You can also describe the mapping of data warehouse sources to targets.
Most organizations have an information architecture that resembles a crowded and completely disorganized bookstore. Data is everywhere. Most companies’ data is unorganized and uncataloged, making it very difficult to find what you’re looking for. This is the main problem, the lack of data discoverability, or lack of data usability & this problem is getting bigger and bigger. Organizations can grow from gigabytes to terabytes to petabytes in ten years. In an era where “data is the new oil,” successful businesses must be able to find and use all their data to gain a competitive advantage. The description and search capabilities of metadata management are critical to successfully finding and using that data. Metadata management is also important, as definitions can change depending on the context of the information.
Metadata management enables you to understand and manage your systems, data, and organization as a whole, providing all parts of your organization with the metadata they need to get a consistent view of data across your organization. Only in this way can an organization perform its functions properly and ultimately do things right.
Different types of personas within an organization need to work together if they want to achieve a certain level of data literacy. Data literacy requires teamwork. You need a solution that handles it all and allows data stewards to interact with data users. See how different teams are using metadata management. For example, the governance team may be more concerned with defining and complying with regulations, but must work in partnership with the IT team. The IT team catalogs the physical systems in which information is stored, documents the various controls and security that are in place around that system, and works with the various teams that manage the systems to ensure that all teams are Make sure you are trained on privacy and compliance.
However, you will need to communicate with your compliance team. Look for a metadata management solution with collaborative governance processes such as workflow, stewardship, version control, and audit trails. Much of the metadata is now segregated across multiple applications and systems. This breaks the link between metadata silos. Some companies use several applications for ETL and store metadata accordingly. There are other applications that focus on data governance and store that data accordingly. There are other applications that store data catalog information and store it separately. All this information is connected and needs to be brought together in one place for better integration, consistency and control through a comprehensive metadata management solution.
Tools for Metadata Management; Alation, Alex Solutions, ASG Technologies, Collibra, IBM, Infogix, Informatica.
Business users interact with organizational metrics and policies. At nearly 80%, they make up the largest group of metadata consumers, asking questions like regional sales and defining the current billing period.
A business data steward manages business metadata such as glossary terms and business rules. These support the generation and consumption of metadata. System data stewards manage technical metadata and its alignment with systems and business rules.
Data architects connect business metadata with technical metadata through conceptual and logical models. They are his second largest group at his 70% of metadata users and ask questions like approved data structures for storing customer data and reporting table sources. The job of a data engineer involves working with metadata and data types. These support the generation and consumption of metadata.
The Need for Metadata Management
When metadata is not managed throughout the lifecycle of a system, organizations create inconsistent metadata silos that don’t meet all of their team’s needs, delivering conflicting information. Users don’t know how much they need to trust the data because it’s not the metadata detailing when and how it got into the system and what business rules were applied. Cost must also be considered. Without effective metadata management, every development project must go through the tedious process of defining data requirements, increasing costs and reducing efficiency. Users are presented with many tools and technologies that lead to redundancy, additional costs, and the data they are looking for is not available, preventing them from fully realizing the value of their investment. Storage costs are high because data definitions are replicated across multiple systems. As your organization matures and adds systems, you need to consider how you manage metadata (rather than just data). Metadata management has clear benefits for business and technical users, and for the organization as a whole.
AI & Its Role in Metadata Management
Edge devices, IoT, and AI are changing metadata management. There is a growing need to leverage this metadata to extract additional value from data. The impact of metadata on production (and productivity) will increasingly depend on its various types of cataloging, mapping, data modeling, machine learning, and edge computing.
Anyone who can successfully operate metadata in these areas will benefit from metadata management. Built-in artificial intelligence (AI) and machine learning (ML) algorithms facilitate metadata classification and data lineage (horizontal, vertical, regulatory). Deliver the data context, consistency, and control you need to achieve the highest efficiency, highest performance, and smartest decisions across all teams and departments.
Conclusion
Implementing metadata management can be simple or complex, depending on how you approach your use case. Data governance and data analytics have been identified as key use cases for metadata management solutions. Metadata management should be aligned with your data governance and data analytics strategy, as users and sources of metadata vary widely. Data governance is a key factor in creating and managing metadata. Data governance works together with metadata management to ensure access to trusted data that is well understood and used in the right context throughout its lifecycle.
Automation and self-service only work when high-quality, trusted data with a shared understanding of metadata is available. The complexity of implementing metadata management depends on the source, use case, users, and size and type of their role. It is also influenced by technologies that generate new sources and use cases and ways to manage them better. Implementing metadata management requires consistently retrieving, storing, and managing metadata at all three levels. Metadata linking between levels and with top-level domains (customers, suppliers, products, etc.) supports search, navigation, and drilldown. The process manages all levels of changing, validating, validating and authenticating conditions. It also requires enterprise-level policy management to ensure high-quality metadata at all levels. Metadata management helps increase business value, improve innovation and collaboration, and reduce risk. It also gives data citizens access to high-quality, trusted data so they can use the right data to deliver accurate insights.